{{serverconnectlatestnews.data.showlatestnews.data[0].news_title}}
{{serverconnectlatestnews.data.showlatestnews.data[0].news_date.formatDate("d MMM yyyy")}}
{{serverconnectlatestnews.data.showlatestnews.data[0].news_teaser}}
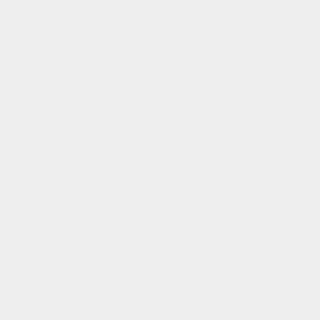
Reducing energy consumption and material loss in steel production using predictive machine learning.
This project has received funding from UKRI as part of Made Smarter Innovation: Sustainable Smart Factory Competition
In 2020, global steel production totalled 1,860 million tonnes, leading to the emission of over 3 billion tonnes of CO2, roughly 8% of all man-made greenhouse gas emissions. Steel producers are facing increasing pressure to reduce the CO2 emissions associated with steel production, both from governments and from industrial consumers.
One aspect of this is to apply machine learning (ML) methods to analyse sensor data in steel plants, and optimise the process parameters. A steel plant can have hundreds of thousands of sensors, providing information related to some 5,000+ process parameters. There is an opportunity to employ data-driven approaches to optimise these processes, increasing productivity, reducing energy consumption, and reducing wasted material.
This project is a collaboration between:
This project aims to deliver data-driven process optimisation for the Electric Arc Furnace (EAF), reheat and hot-rolling. The project will establish the data pipelines to extract, transform and load process parameters, and develop and train machine learning algorithms to analyse these data. This will result in real-time actionable insights to optimise Electric Arc Furnace process parameters and reheat/hot-rolling scheduling, enabling savings in energy consumption and material loss.
Dates - 1st September 2022 – 31st August 2024
Sponsor - Innovate UK
Project Value - £1,955,344
Funding - £977,509